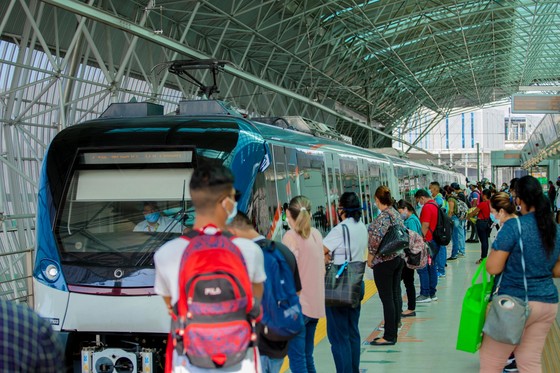
Mastria: Mobility Orchestration in the digital age
Transport congestion of any kind of flow (cars, trains, bicycles, e-scooters, pedestrians, passengers...) is a major challenge in large urban zones. Considering the interdependency of transport modes in today’s dense urban environments, an incident on one mode of transport might create a series of reactions that will impact all the city’s transportation system globally. To respond to this challenge, Alstom is developing Mastria , the first multimodal supervision and mobility orchestration solution. In this article, we will present the global concept as well as two different Mastria functions in their operational environment and how their implementation led to an improvement in the management of daily operations in public transport systems (both road and rail based). Further, we will show how the implementation of these functions into an aggregated mobility orchestration is progressing.
About Mastria
Mastria supervises city mobility in order to detect and report real-time information from all transport modes to the operators’ control centers and provides recommendations on which procedure to follow and how to implement corrective actions in real time from the city mobility operation center. The goal of Mastria is to maximise fluidity between all the means of transportation in a city and to orchestrate passengers journeys. Mastria is a software solution only, in order to remain agile and be adaptable to any kind of OCC and traffic supervision tools already in place. Mastria can therefore be combined in a city using Alstom operation control solutions, but also those of any other manufacturer.
Mastria will help analysing, simulating and predicting mobility demand thanks to multiple data sources such as telecommunications (global demand monitoring mobile phones data), ticketing (via ticket gates), counting systems (on platforms or vehicle doors), CCTV, bluetooth beacons and heterogenous data (weather, traffic, incidents) that have an impact on mobility demand. Through big data integration, Mastria monitors people flows in real-time and predicts impacts at the same time to anticipate demand evolution, analyse modal shifts and hub flows.
The objective of Mastria is to help authorities and operators to adapt the transportation offer to the actual demand and synchronize better the different modes of transportation. In a context where private initiatives are driving the development of Mobility as a Service (MaaS) into an individual approach, authorities want to control and regulate the transportation systems of the city in order to promote sustainable mobility.
For example, the weather has a strong impact on the usage of various transport modes. Bad weather can bring people into the metro and connected bus services shall therefore be reinforced; in this case, regular users of the public transport offer could be warned to advance or postpone their trip in order to avoid congestion. On the opposite side, during good weather, free-floating scooters shall be disposed at the exit of the main regional train stations. Mastria can predict the demand evolution and give advice to authorities and operators to act accordingly as well as recommend the best way to move to people around the city.
Alstom decided to join their efforts with urban operators on specific use cases to implement Mastria functions step by step and thus provide the market with an all-encompassing mobility orchestration solution. Indeed, as with any product development, intermediate steps need to be taken before the final product is operational. In the following, two use cases will therefore be presented. The first one focuses on the management of heterogeneous bus system, the second one on a metro connection optimization.
Zaragoza, how to coordinate multiple operators
To improve Zaragoza’s metropolitan bus network management, CTAZ (Transport Consortium of Zaragoza Area) and Alstom in Spain developed a pilot project to implement Mastria solution. The ultimate objective is to resolve incidents more quickly in real time and coordinate the actions taken by the authority and the operators in a more efficient manner.
The fleet of buses coordinated by CTAZ on which the innovation project is based includes 6 different operators and covers 30 municipalities, with a total of 25 lines. The organization of this complex network, which annually transports over 5 million passengers, represents a real challenge, as the different operators have diverse internal management mechanisms. Indeed, CTAZ which is the entry point for the passenger to get information about the bus traffic struggled to monitor the incidents encountered by the different operators. By not knowing what is happening on its network, the authority cannot inform passengers accurately . Before the implementation of Mastria, the monitoring of the different incidents was done manually by the authority only if a customer would raise an issue by calling the authority. Further quality of service was monitored on a monthly basis but not in real time. Taking corrective action was therefore very time consuming.


Panama, metro optimization use case
Another city in which Mastria has proven itself is Panama City: here, the application domain and results are more quantitative. Mastria is used to relieve some transport hubs from overcrowding and platform saturation.
The Panama Metro consists of two lines, which link the north and east sides of the metropolitan area to the city center. The 15.8-kilometer Line 1, opened in April 2014 serves fourteen stations, with a fifteenth station planned. Line 2, opened in April 2019, covers a 21-kilometer route and serves sixteen stations, with San Miguelito being the interchange station for both lines. The Panama Metro was built as a turnkey project by Alstom in order to relieve the road traffic congestion between Panama City and the suburb of San Miguelito, and to offer commuters a viable alternative to road transport, as the Metrobus transport system is suffering multiple issues.
When the second metro line in Panama was opened, it was quickly a victim of its own success with overcrowded platforms, queues in the staircase etc. Due to a ridership higher than expected, a huge saturation was generated at the interchange station of San Miguelito (see picture below). The operator has tried to implement operational solutions to reduce saturation, such as sending empty trains or not stopping at all stations. But there was no way of measuring the impact of these measures on station overcrowding. The challenge was to measure and anticipate the saturation effect and find the best solution to reduce the impact on passenger travel time.




Towards a multimodal integration
The next step for Panama transport authority is to extend such system to a multimodal platform to better integrate the buses with the metro and thus make multi-modal operations more manageable. The first step will be to analyse, monitor and model global mobility in the city. Then, it will be possible to synchronize services, adapt transport offer to the demand and inform efficiently in real-time the passengers.
The objectives of the multi-modal platform are to:
- support the Planning the future lines of Metro
- Test different services scenarios offline for planning and preparing action plans for mobility orchestration
- Optimize operations in real-time depending on the current situation
- Predict and react efficiently to incidents
Conclusion
With the multitude of transport offers and actors today, it has become more complicated for municipal transport authorities to implement transport policies due to a loss of control. By helping to better understand the mobility of its citizens, Mastria is giving the tools to transport authorities to regain control of their transport systems and organize integrated mobility on their territory in an orderly manner. Based on artificial intelligence and modelling simulation, the force of Mastria is that it leverages existing big data from public transportation (services, vehicles, ticketing), road (traffic information, parking occupancy), telecoms & IoT (origin destination matrix, heat maps) and social factors (social networks, weather, city sensors).
At the final stage, Mastria aims to:
- Promote sustainable transportation by increasing public transport ridership
- Improve the planning for infrastructure investments thanks to a better understanding of citizens mobility and the simulation of development scenarios
- Implement a multimodal integration: Analyse modal shift and network bottlenecks, Business Intelligence for people flow fluidity, Hub Management
- Enable Mobility as a Service by generating high quality information accessible through open data platforms for on-demand mobility services
- Support operational decision-making support by predicting passenger’s behaviour in real-time but also by informing efficiently the public and a quick reaction for incident solving
